Multi-Modal Processing of Speech and Language: How-to Videos and Beyond
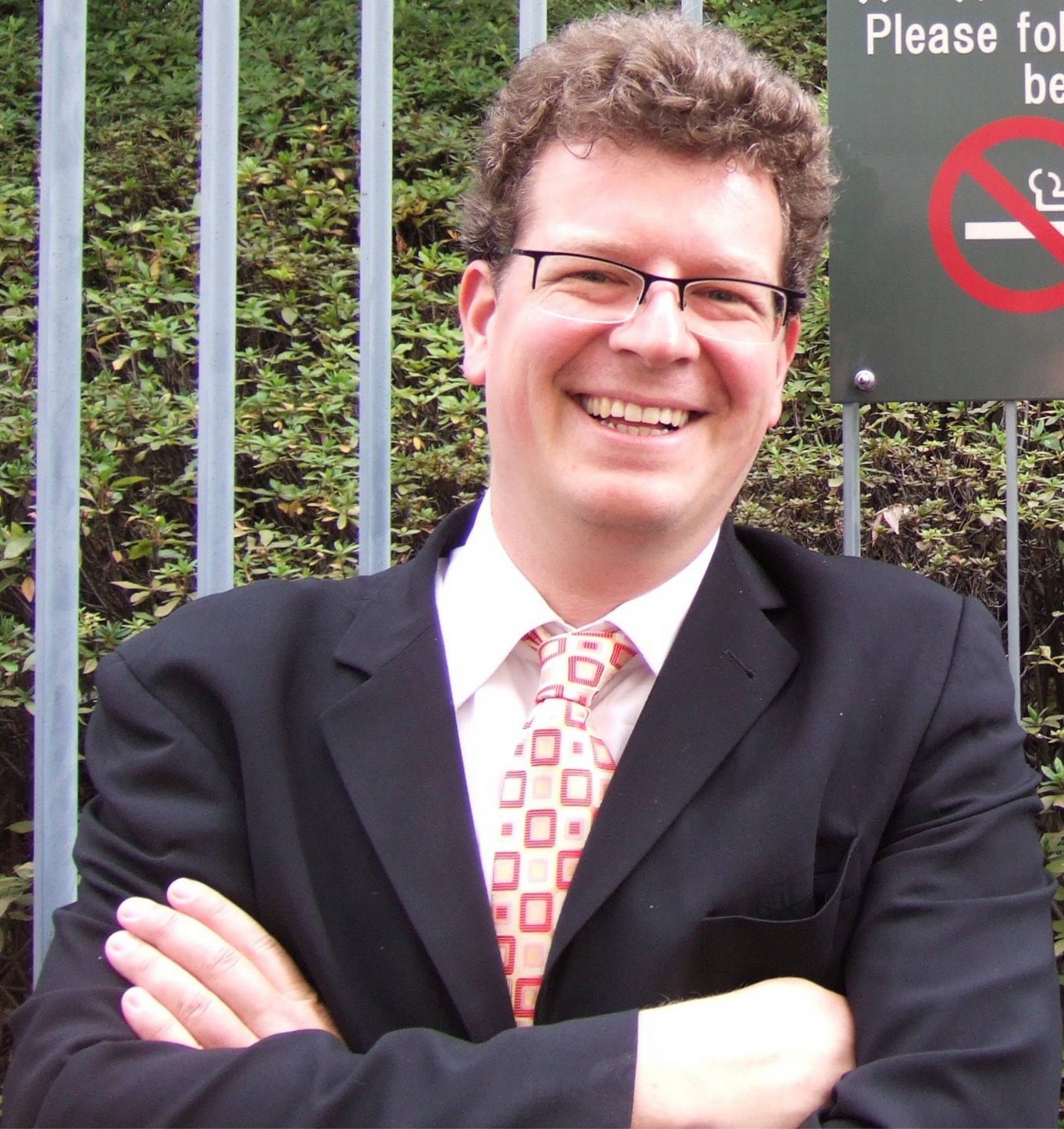
Florian Metze
https://www.cs.cmu.edu/~fmetze/interACT/Home.html
Sunday, December 15, 14:30-15:30
[Slides]
Abstract: Human information processing is inherently multimodal. Speech and language are therefore best processed and generated in a situated context. Future human language technologies should therefore be able to jointly process multimodal data, and not just text, images, acoustics or speech in isolation. Despite advances in Computer Vision, Automatic Speech Recognition, Multimedia Analysis and Natural Language Processing, state-of-the-art computational models are not integrating multiple modalities nowhere near as effectively and efficiently as humans. Researchers are only beginning to tackle these challenges in “vision and language” research, e.g. at the 2018 JSALT workshop. In this talk, I will present recent work on multi-modal processing (recognition, translation, and summarization) of how-to videos and lectures, and show the potential of multi-modal processing to (1) improve recognition for challenging conditions (i.e. lip-reading), (2) adapt models to new conditions (i.e. context or personalization), (3) ground semantics across modalities or languages (i.e. translation and language acquisition), (4) training models with weak or non-existent labels (i.e. SoundNet or bootstrapping of recognizers without parallel data), and (5) make models interpretable (i.e. representation learning). I will present and discuss significant recent research results from each of these areas and will highlight the commonalities and differences. I hope to stimulate exchange and cross-fertilization of ideas by presenting not just abstract concepts, but by pointing the audience to new and existing tasks, datasets, and challenges.
Biography: Florian Metze is an Associate Research Professor at Carnegie Mellon University, in the School of Computer Science’s Language Technologies Institute. His work covers many areas of speech recognition and multimedia analysis with a focus on end-to-end deep learning. Currently, he focuses on multimodal processing of speech in how-to videos, and information extraction from medical interviews. He has also worked on low resource and multilingual speech processing, speech recognition with articulatory features, large-scale multimedia retrieval and summarization, along with recognition of personality or similar meta-data from speech.
Conversational Machines: Towards bridging the chasm between task-oriented and social conversations
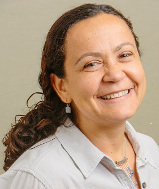
Dilek Hakkani-Tür
https://www.linkedin.com/in/dilek-hakkani-tur-9517543
Monday, December 16, 13:30-14:30
Abstract: Conversational systems are typically considered in two categories, task-oriented dialogue systems and social bots. Task-oriented systems aim to help users accomplish a specific task through multi-turn interactions, whereas socialbots focus on enabling engaging and natural open-domain conversations. In real-user interactions, even when the conversation participants have a task or goal in their mind, they can easily say things that fall out of the boundaries of that task domain, and vice versa. The ability to engage in knowledgeable social interactions and gracefully transition back and forth to the task is crucial for enabling natural conversations. In this talk, I’ll summarize our recent work in both fronts. Starting with task-oriented interactions, I’ll focus on bootstrapping task-oriented dialogue systems from simulated seeker-provider conversations, creation of a unified dialogue act schema to benefit from previous research and datasets, and dialogue state tracking. This will be followed by a summary of learnings from previous Alexa Prize challenges and progression of our work.
Biography: Dilek Hakkani-Tür is a senior principal scientist at Amazon Alexa AI focusing on enabling natural dialogues with machines. Prior to joining Amazon, she was leading the dialogue research group at Google (2016-2018), a principal researcher at Microsoft Research (2010-2016), International Computer Science Institute (ICSI, 2006-2010) and AT&T Labs-Research (2001-2005). She received her BSc degree from Middle East Technical Univ, in 1994, and MSc and PhD degrees from Bilkent Univ., Department of Computer Engineering, in 1996 and 2000, respectively. Her research interests include conversational AI, natural language and speech processing, spoken dialogue systems, and machine learning for language processing. She has over 70 patents that were granted and co-authored more than 200 papers in natural language and speech processing. She is the recipient of three best paper awards for her work on active learning for dialogue systems, from IEEE Signal Processing Society, ISCA and EURASIP. She served as an associate editor for IEEE Transactions on Audio, Speech and Language Processing (2005-2008), member of the IEEE Speech and Language Technical Committee (2009-2014), area editor for speech and language processing for Elsevier’s Digital Signal Processing Journal and IEEE Signal Processing Letters (2011-2013), and served on the ISCA Advisory Council (2015-2019). She is the Editor-in-Chief of the IEEE/ACM Transactions on Audio, Speech and Language Processing, and a fellow of the IEEE and ISCA.
End-to-End Speech Synthesis
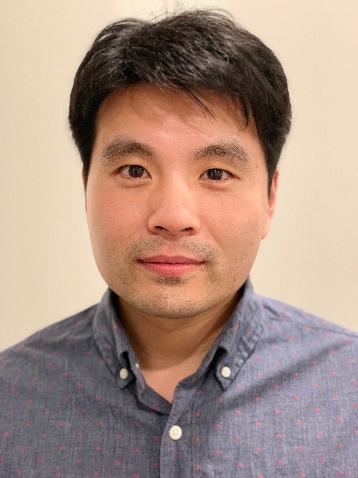
Yuxuan Wang
https://www.linkedin.com/in/yuxuan-wang-85b5704
Monday, December 16, 14:30-15:30
[Slides]
Abstract: A text-to-speech (TTS) synthesis system typically consists of multiple components. Building these components often requires extensive domain expertise and may contain brittle design choices. In this talk, I will describe recent advances in end-to-end neural speech synthesis. In particular, I will first discuss Tacotron & Tacotron 2, core end-to-end TTS models that can synthesize high-quality speech directly from text inputs. These models can be trained from scratch, greatly simplifying the voice building pipeline. Then, I will talk about how these models could help tackle key challenges in the field, such as prosody and speaker modeling, which is important to enable expressive and personalized TTS. Finally, I will also discuss the opportunities and challenges for end-to-end TTS and review some recent relevant work.
Biography: Dr. Yuxuan Wang is a Director at ByteDance AI Lab, where he leads research & development in speech, audio and music intelligence. Before that, he was a research scientist at Google AI. He received his Ph.D in computer science at the Ohio State University as a Presidential Fellow. He has published more than 60 papers, including several influential works in deep learning based speech processing. Notably, he built the first DNN based speech enhancement system and end-to-end neural speech synthesis system known as Tacotron, both of which have been widely adopted in academia and industry. His interests include sound understanding & synthesis, intelligent music processing, machine learning, etc. and their use cases in real-world products.
The Deep Kinship between Speech and Music
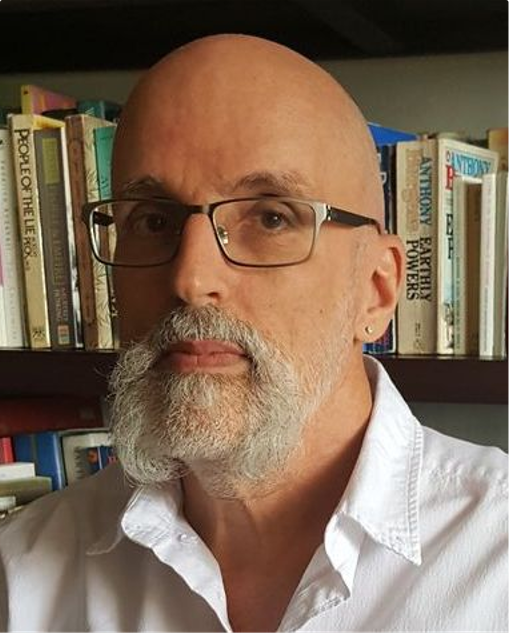
Lonce Wyse
Tuesday, December 17, 14:30-15:30
[Slides]
Abstract: Speech and music are uniquely human capabilities. They bear a kinship to each other superficially since they are both sound-based, and more deeply because they share much of the biological, mechanical, and neurological resources that have evolved in humans for their perception and generation. No matter what lens we use to focus our gaze – cultural, evolutionary, neurobiological, computational – we find deeply entangled similarities and differences between the two phenomena that inform our understanding of them, as well as how we design and engineer models that exhibit similar capabilities. In this talk I will reflect on some of these relationships and how they have been, and can be productive for modeling with particular attention on notions of content and style.
Biography: Lonce Wyse is an Associate Professor with the Department of Communications and New Media at the National University of Singapore, and directs the Arts and Creativity Lab of the Interactive and Digital Media Institute at NUS. He holds a PhD in Cognitive and Neural Systems (Boston University, 1994), and was a Fulbright Scholar the following year at Taiwan National University. He serves on the editorial boards of the Computer Music Journal (MIT Press), Organized Sound (Cambridge University Press), and the International Journal of Performance Arts and Digital Media. Lonce’s current research focus is on developing neural networks as interactive sound synthesis models. Other research endeavors include sound perception, real-time musical communication, improvisation, and notation, and networked music making. He teaches courses on software studies, creative coding, media art, and interactive audio.
Mechanisms of Robust Speech Recognition in the Human Auditory Cortex
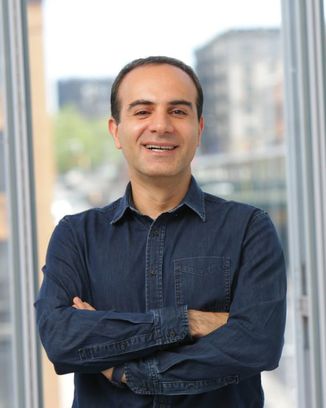
Nima Mesgarani
Wednesday, December 18, 12:00-13:00
[Slides]
Abstract: Speech perception in real-world situations requires a listener’s auditory system to extract and represent linguistic features often in the presence of interfering sound sources and changing background conditions. This complex process includes nonlinear, dynamic, and adaptive transformations of the speech signal as it propagates through the auditory pathway. The behavioral consequence of these neural processes is the remarkable human ability to perceive speech in adverse acoustic conditions. Despite the immense importance of this research question, the nature of these transformations in the human brain remains unclear. The progress is lacking because cortical processing of speech is highly spatially precise, temporally feeling, and computationally nonlinear, far beyond the resolution of noninvasive methods and the capacity of common computational models. To address these shortcomings, we use invasive human electrophysiology and deep learning models to determine the where, when, and how of speech processing in the human auditory cortex. This talk reports progress in three areas of research: I) characterizing the adaptive and dynamic properties of speech representation in the human auditory cortex that enable robust speech recognition, II) Neural decoding of who a person wants to listen to, which aims to create a mind-controlled hearing aid that tracks the brain-waves of a listener to identify and amplify the voice of a target speaker in a crowd. and III) More accurate computational models of the transformations that the brain applies to speech at different stages of the auditory pathway. Together, this combination of experimental and computational approaches advances our knowledge of the functional organization of human auditory cortex and pave the way toward more complete models of cortical speech processing in the human brain.Biography: Nima Mesgarani is an associate professor at Electrical Engineering Department and Mind-Brain-Behavior Institute of Columbia University in the City of New York. He received his Ph.D. from the University of Maryland and was a postdoctoral scholar in Center for Language and Speech Processing at Johns Hopkins and the Neurosurgery Department of the University of California San Francisco. He has been named a Pew Scholar for Innovative Biomedical Research and UNICEF-Netexplo top-10 innovator of the year. He has received the National Science Foundation Early Career and Auditory Neuroscience Young Investigator Awards. His interdisciplinary research combines theoretical and experimental techniques to model the neural mechanisms involved in human speech communication which critically impacts research in modeling speech processing and speech brain-computer interface technologies.